What can be done to improve weather prediction and load forecasts?
Weather forecast error, whether large or small over a year, can result in substantial added costs to energy organizations. With today's movement toward real-time pricing and sub-hourly markets, it is critical to be as accurate as possible with load forecasts.
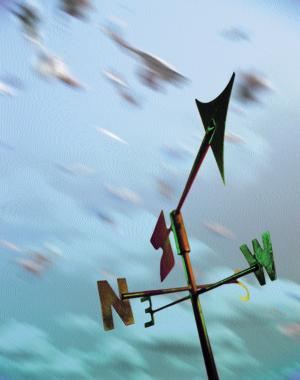
Short-term load forecast error (i.e., percent variations high and low within a four-day period) typically is caused by load measurement error, weather forecast error, and load forecast model error.
Benefits of improving the day-ahead weather and load forecast by just 1-degree Fahrenheit include:
- About $20 million to $25 million per year for a Northeast regional transmission authority; and
- About $1 million to $2 million per year for a large regional distribution company.
Con Edison reported that its earnings per share in 2003 vs. 2002 were positively affected by $0.15 per share. Earlier it reported -$.05 earnings per share due to weather effects-demonstrating that over a short period of time, earnings can swing from -$11 million to +$36 million-a $47 million shift.