Benefits and drawbacks of the most popular estimation methods or modeling techniques.
Christopher Athaide is a partner at the energy analytics firm Cygnet Risk Group Ltd. He can be reached at cathaide@ cygnetriskgroup.com.
When one tries to model a system, several variables can affect the system’s behavior. The modeler tries to extract a minimal subset of variables that can satisfactorily explain most of the effects under study: in this case, a natural-gas storage facility (NGSF). The question we would like to answer is: What is its value over a fixed time period?
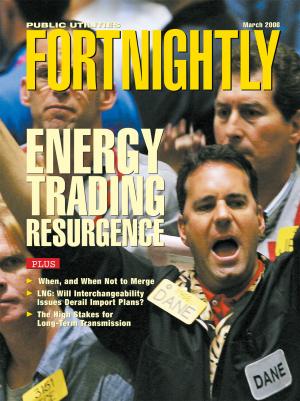
To determine this, we must identify the attributes, or inputs, that are the key determinants of this value, and then determine how the value changes as a function of the inputs.
Before we go about trying to value natural-gas storage, we should try and come up with a list of important considerations in any valuation process. The valuation method should use:
- Forward prices; and
- Implied volatilities (instead of historical volatilities);
The method should be able to capture the intricacies of most NGSFs, including:
- Injection/withdrawal ratchets: In this case, injections and withdrawals depend on inventory levels;
- Time-based injection/withdrawal and inventory constraints;
- Injection/withdrawal fuel factors and commodity rates; and
- Ad Valorem taxes.
Also, the data requirements should be modest, and the calculation time should be reasonable.
The five main methods for valuing storage are: