Each DR portfolio will have a different set of AMI needs, based on overall technology infrastructure.
Ross Malme is president and CEO of RETX Energy Services Inc. and the operating agent for the International Energy Agency’s Demand Side Management Programme’s Task XIII. He also is immediate past chairman of the Peak Load Management Alliance. Contact him at rmalme@retx.com. Daniel Violette is CEO of Summit Blue Consulting and a principal in the strategy practice. Rachel Freeman is a consultant at Summit Blue Consulting. Contact Violette and Freeman at (720) 564-1130. Pete Scarpelli is vice president of business development at RETX Energy Services Inc. and the inventor of RETX’s ePath Load Management Dispatcher product.
Advanced metering infrastructure (AMI) evaluations will benefit greatly from creating an appropriate DR portfolio as part of the overall solution.
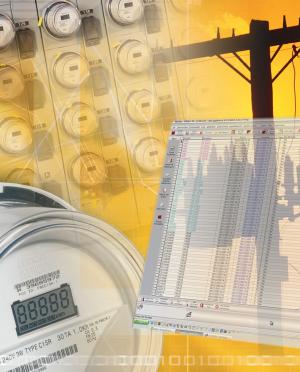
In the Energy Policy Act of 2005 (EPACT), Congress sent a strong message to electric utilities, consumers, and industry regulators that they need to get serious about advanced metering, time-based rates, and demand response (DR).
To underline this point, EPACT states:
“It is the policy of the United States that time-based pricing and other forms of DR, whereby electricity customers are provided with electricity price signals and the ability to benefit by responding to them, shall be encouraged, the deployment of such technology and devices that enable electricity customers to participate in such pricing and DR systems shall be facilitated, and unnecessary barriers to DR participation in energy, capacity and ancillary service markets shall be eliminated.”
However, a number of state public utility commissions (PUCs) and electric utilities were evaluating the implementation of advanced metering infrastructure (AMI) even before EPACT was signed into law. In many cases, a significant portion of the economic justification for installing AMI systems is attributed to potential benefits derived from DR applications. AMI can be an enabling technology for DR efforts, and including DR benefits in the AMI business case is both reasonable and prudent.