Forecasting brings wind energy under control.
Michael T. Burr is Fortnightly’s editor-in-chief. Email him at burr@pur.com.
Advancements in forecasting have improved the reliability of day-ahead and hour-ahead estimates of wind generation. Wind never will behave like a base-load power plant. But as system operators integrate wind forecasts into their planning and market processes, they’re transforming intermittent wind energy into a variable but reliable resource.
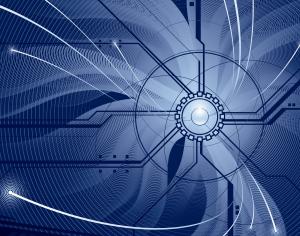
When transmission system operators think about wind power, two problems leap to mind. First, wind resources tend to be located far away from load centers, so new wind farms sprouting up across the countryside will require new long-distance transmission lines. Second, for purposes of system operations, wind is an “intermittent” source of power—unpredictable and non-dispatchable. As a result, operators have viewed wind power as an energy-only resource, essentially useless (and sometimes detrimental) for system reliability and security.