The efficient frontier is a portfolio analysis concept designed to assess risk vs. return for an investment portfolio.
James Letzelter is a partner at Webb, Scott & Quinn Inc. Contact him at Jim_Letzelter@wsqi.com.
The efficient frontier is a portfolio analysis concept designed to assess risk vs. return for an investment portfolio. While the financial projections of individual assets are key to the analysis, the end result is critical to successful structuring of the portfolio as a whole. Key to the efficient frontier is that it represents the highest level of a portfolio's return for any given level of risk. It can be applied to physical assets, as well as financial instruments—simultaneously. Figure 1 displays a typical efficient frontier chart. The horizontal axis represents risk (typically in the form of standard deviation of return in dollars), while the vertical axis represents return (typically in the form of the mean return expected of the portfolio). The line, which is the efficient frontier itself, represents the highest level of expected return for any given level of risk for any possible combination of assets for the portfolio. The area under the curve is populated by every other "non-efficient" portfolio that can exist.
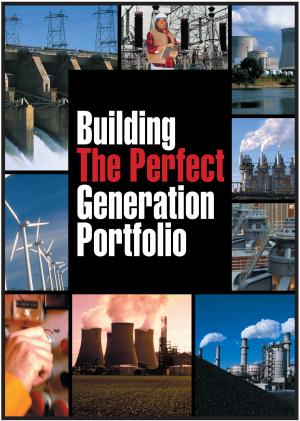
Plotting a power generation portfolio is an extremely useful approach to understanding risk and return—and the power industry is well suited for it. For one, power markets are generally volatile—even in the long term. Recent poor financial performance by individual assets-and portfolios as a whole-has highlighted the need for more insightful approaches to asset acquisition and portfolio structuring.