Simulation modeling can improve O&M and capital-planning processes.
Patrick J. Delaney is vice president, analytic solutions at Davies Consulting Inc. (DCI). Wiko Kabiling is an analytic consultant at DCI, specializing in statistical analysis and mathematical modeling.
Electric utilities are faced with the challenge of managing a range of aging distribution assets that are critical to system reliability. They also are threatened with potentially huge costs as they seek to replace these assets over the coming years to maintain reliability. Making intelligent decisions about asset maintenance and replacement requires accurate information about the failure patterns of these assets over time. However, most data elements that could shed light on such patterns—asset condition, joint use, maintenance patterns, or results of stratified inspection—are not widely available. Still, utilities must forecast capital and O&M spending requirements each year, regardless of their understanding of such asset failures.
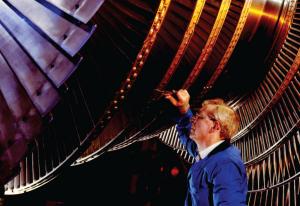
In addition to these gaps in data, a further lack of tools and processes make it difficult to support budget-allocation decisions. For the most part, capital funding decisions are being made using the simple but potentially inaccurate forecasting method of taking the average of asset failures over a certain period of time. Existing replacement or maintenance strategies don’t necessarily match the costs and reliability that will be experienced in several years.