Customer-specific demand-response strategies become more sophisticated.
Chris McCall is a senior project manager and Heber Weller is a smart grid program manager with SAIC. Andrew Thomas is senior vice president of operations at World Energy Solutions.
Demand response (DR) is one of the fastest growing and most enthusiastically received programs in the smart-grid space, with Barclays Capital predicting it could grow to a $20 billion market by 2020. But to reach that level, both the market for participating in DR and the systems for executing it need to become more efficient.
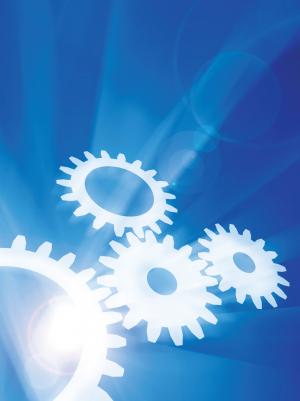
DR is a series of programs typically sponsored by the local utility, independent system operator (ISO) or retail energy provider. The most common DR structure pays electric customers to be on call to reduce electricity usage when the grid is being pushed to its capacity limits. In one form of DR, independent (i.e., non-utility owned) curtailment-service providers (CSPs) work with electric customers to curtail energy usage during times of peak demand, providing a valuable service to grid operators—one that can prevent blackouts and reduce the need for building new power plants. In return for this service, operators pay a set price per megawatt to CSPs, who in turn pass on a portion of this payment to their customers.