Price forecasting is a significant business process within any energy merchant that trades electricity and natural gas. Business planning, trading, mergers and acquisitions (M&A), even rate-case activities rely upon some type of a price forecast as the foundation to analysis.
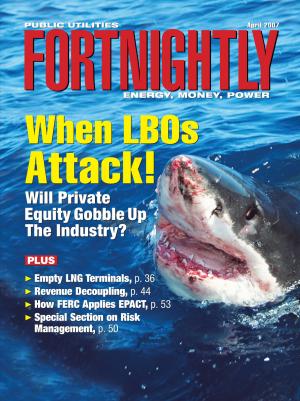
The problem with a single forecast is that it never is correct. As soon as the forecast is complete, the world changes and the information becomes dated and often even irrelevant.
This article reviews two common approaches used to forecast prices and introduces the concept of “three-dimensional price forecasting.” Incorporating these other dimensions changes the environment in a structural way.
Econometric vs. Fundamental Forecasting Methods
Energy-price forecasting employs two families of analysis. One is grounded in market based econometrics; the other relies on supply-and-demand fundamentals. Each method requires a deep set of experience and knowledge in very different areas.