Why predictions from the Energy Information Administration may contain systematic errors.
Tim Considine is a member of the Energy and Mineral Engineering Department. Frank Clemente is a senior member of the graduate faculty at Penn State and former director of the university’s Environmental Policy Center. Contact Clemente at fac226@psu.edu. The authors would like to express their appreciation for suggestions from Professor Maximilian Auffhammer (UC-Berkeley).
The difficulties of predicting future trends in energy are widely recognized (see Reference4). Even the most sophisticated of forecasting models cannot account fully for a myriad of complex and generally uncontrollable variables. Thus, energy policy-makers necessarily must anticipate a wide range of possible outcomes in formulating energy plans.
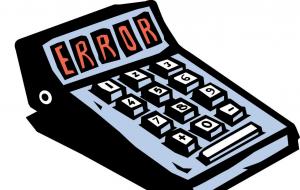
The issue here, however, is not how difficult it is to predict energy prices, supply, and demand. Our question, rather, is whether systematic biases are built into forecasts, causing them to err repeatedly in the same direction. And the more visible the forecast (and the more likely also that it will be used), then the more likely it is that the error will be compounded in a variety of settings.
In the case of the U.S. Energy Information Administration (EIA), for example, natural gas (NG) data and projections are used widely in regulatory proceedings, energy planning, scientific research, investment decisions, litigation, and legislation. In such cases, systematic bias can have profound socioeconomic implications—not only within the United States but in other nations as well. Indeed, the National Energy Board of Canada regularly includes EIA NG forecasts in its projections. Even OPEC scholars use EIA projections as a benchmark in their research.