Intelligent networks support better decision making.
Rick Nicholson is vice president of research with Energy Insights.
Sophocles once said, “Quick decisions are unsafe decisions.” Apparently Sophocles did not work in the utility industry. Utilities must make quick decisions every day to maintain a safe and reliable grid. As they have learned, the key to a quick and safe decision is making a well-informed decision. Yet utilities face challenges in providing enough information for their employees and automated systems to make these types of decisions. To address this challenge, some utilities are looking at intelligent grid analytics to help them more quickly process large amounts of complex data and make better-informed decisions.
Defining Analytics
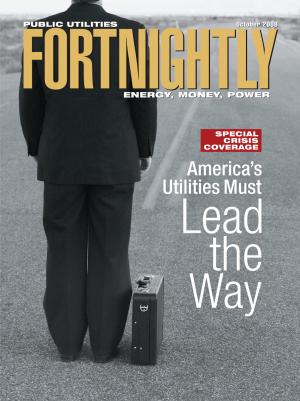
At their most basic level, analytics applications are fact-based decision-support systems that turn data into information that a company can use to make a decision or take action. According to IDC, analytics applications include: query, reporting and analysis software - ad hoc query and reporting tools, production reporting tools, multidimensional analysis tools, and software dashboards; and advanced analytics— software that uses advanced technologies to discover relationships in data and make predictions that are hidden, not apparent, or too complex to extract using query, reporting, and analysis software.