Effective metrics give solar its due credit.
Richard R. Perez (perez@asrc.cestm.albany.edu) is a senior research associate with the Atmospheric Sciences Research Center at SUNY-University at Albany. Mike Taylor (mtaylor@solarelectricpower.org) is director of research at the Solar Electric Power Association. Thomas E. Hoff (tomhoff@cleanpower.com) is founder and principal of investment consulting firm Clean Power Research. J.P. Ross (jpross@sungevity.com) is a vice president with home-solar energy service provider Sungevity.
Photovoltaic (PV) power generation is an intermittent, non-dispatchable resource generally considered as energy-only with no capacity credit. However, there is ample evidence that solar energy reliably is available at peak demand time when loads are driven by day-time commercial air conditioning, and can contribute effectively to increasing the capacity available on a regional grid.
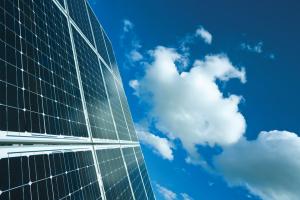
Several studies have shown that the availability of solar power plants often is high during times of peak electrical demand when peaks occur in summer and are driven by day-time commercial air conditioning. These peaks are intensified during heat waves, which are fueled by solar gain. Thus, the resource creating the demand also can be used to meet that demand and provide local grid decongestion. This relieves load pockets and transmission bottlenecks, providing the equivalent of firm peaking capacity, i.e., effective capacity.