Evaluating the impact of dynamic pricing.
Trevor R. Roycroft, PhD, is an independent consultant providing economic and policy research and analysis. Email him at trevor@roycroftconsulting.org.
Dynamic pricing will expose retail consumers to new opportunities and challenges in electric power markets. The impact of dynamic pricing on low-income consumers deserves special attention as low-income populations have different load profiles, resulting in different abilities to respond to price signals as compared to higher income households.
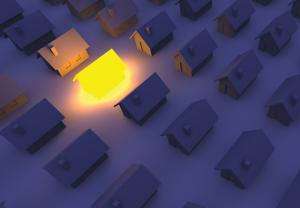
In the November 2010 Fortnightly article “Dynamic Pricing and Low-Income Customers” by Lisa Wood and Ahmad Faruqui (the “Wood/Faruqui article”), the authors conclude that low-income customers have much to gain from dynamic pricing.1 Given the challenge of transitioning low-income consumers into dynamic pricing, the support for this conclusion deserves full evaluation.