The changing architecture of demand response in America.
Ahmad Faruqui, Ryan Hledik and Sanem Sergici are economists with The Brattle Group. Faruqui (ahmad.faruqui@brattle.com) led the multi-company research team (including Brattle Group, Freeman, Sullivan and Co., and Global Energy Partners) that produced the FERC assessment cited in this article. The authors acknowledge research assistance from Lamine Akaba and Jennifer Palmer.
There is universal agreement that demand response (DR) should play an important role in meeting America’s energy needs. And total disagreement on the nature of this role. Two key questions continue to be debated with much vigor in regulatory proceedings before state commissions and in executive boardrooms. First, how big of a role can DR play in meeting future energy needs? And second, what should be the mix of programs in the DR portfolio?
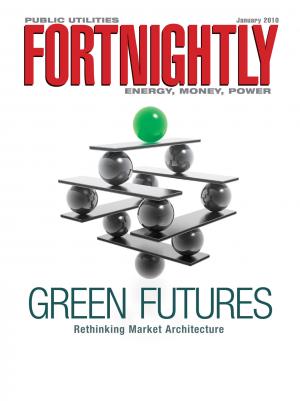
With a view to advancing this debate, the authors present the key findings from a comprehensive assessment of the potential for DR that was submitted by the Federal Energy Regulatory Commission (FERC) to Congress last year. Evidence points toward a major shift in both restructured and regulated states toward dynamic pricing programs. This shift might change the architecture of DR in the country. But before this change can take place, several barriers need to be overcome. Scientific experimentation can play a salient role in this endeavor. However, while many experiments have been conducted and provide significant insights into customer behavior, the results might not be transferable to other regions. There will be an ongoing need for experimentation to guide the development of appropriate public policies and to address customer and regulatory concerns that are unique to each geography.